Anomaly Detection
Anomaly Detection generally finds technical bugs and pinpoint changes that could result from human behavior
Updated: October 9, 2023
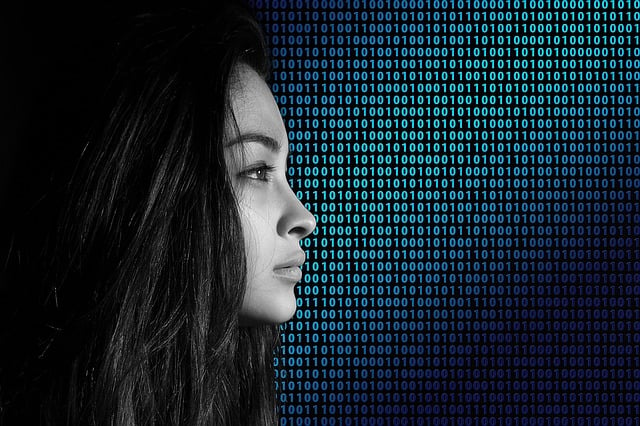
Anomaly detection, also known as outlier analysis is an important part of data mining that identifies information or observations that are significantly different from the overall pattern of behavior in the dataset. It generally finds technical bugs and pinpoint changes that could result from human behavior.
Anomalies or data points that deviate from the norm are more clearly visible when they happen after gathering enough data to form a baseline. Finding anomalies correctly is essential in many industries. Although some anomalies can be false positives, but others signify a larger issue.
Bank fraud and hacking are some of the most commonly identified anomalies in data. Digital forensics software is generally used to detect the unusual behavior. Artificial intelligence (AI) are used by many of these systems now to monitor for anomalies around the clock automatically.
Supervised detection and unsupervised detection are two types of anomaly detection used by companies. Cluster-based algorithms, Neural networks, Density-based algorithms and Bayesian-networks are the most commonly used techniques to find anomalies. However, the type of data used to train the machine, and the organization is continually gathering that determines the detection techniques used to find anomalies.
Preventing data breaches or fraud, finding new opportunities and automating reporting and result analysis are benefits of anomaly detection.